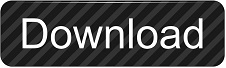
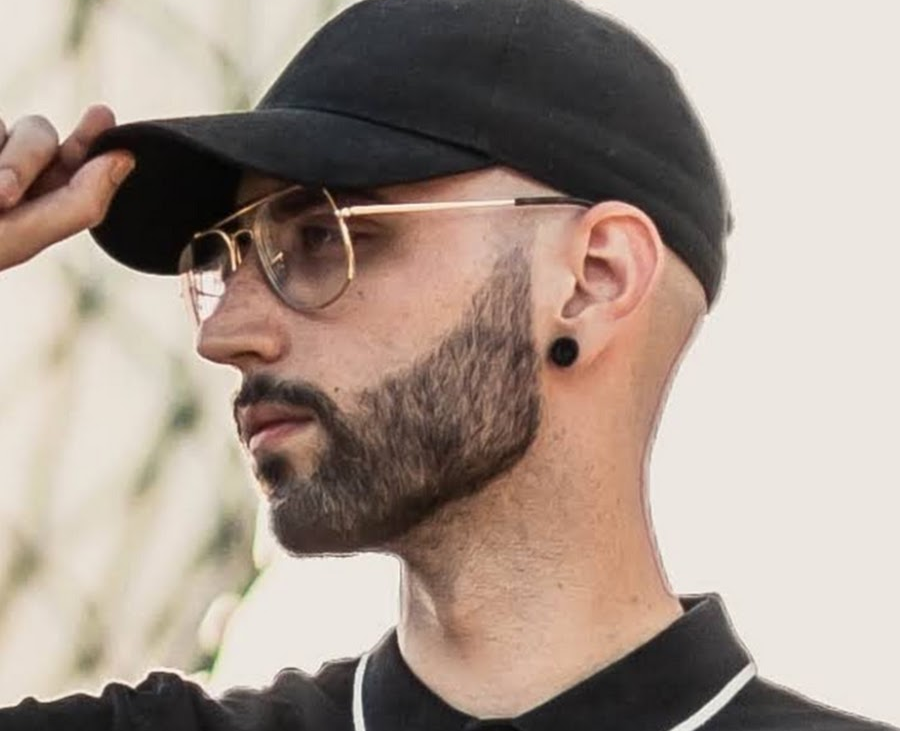
This can mean that researchers miss important features that skew the training of their models. It also muddies the origin of certain data sets. This means that some tools end up being tested on the same data they were trained on, making them appear more accurate than they are. But this meant that many tools were built using mislabeled data or data from unknown sources.ĭriggs highlights the problem of what he calls Frankenstein data sets, which are spliced together from multiple sources and can contain duplicates. Researchers wanted to help quickly, and these were the only public data sets available. Information about covid patients, including medical scans, was collected and shared in the middle of a global pandemic, often by the doctors struggling to treat those patients. Many of the problems that were uncovered are linked to the poor quality of the data that researchers used to develop their tools. “The pandemic has put problems in the spotlight that we’ve been dragging along for some time,” says Wynants. So what went wrong? And how do we bridge that gap? If there’s an upside, it is that the pandemic has made it clear to many researchers that the way AI tools are built needs to change. “I fear that they may have harmed patients,” says Wynants. Wynants and Driggs both say that a few of the algorithms they looked at have already been used in hospitals, and some are being marketed by private developers. Unrealistic expectations encourage the use of these tools before they are ready. “There is a lot of hype about machine-learning models and what they can do today,” says Driggs. But they are concerned that it could be harmful if built in the wrong way because they could miss diagnoses or underestimate risk for vulnerable patients. Wynants and Driggs still believe AI has the potential to help. Incorrect assumptions about the data often meant that the trained models did not work as claimed.
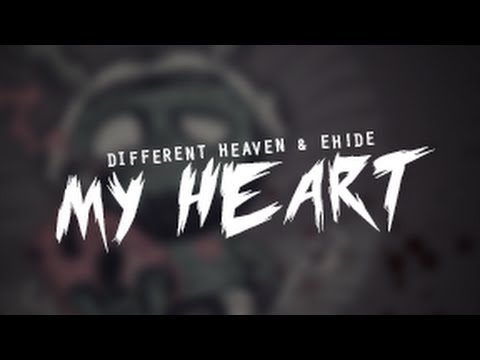
“But I don’t think we passed that test.”īoth teams found that researchers repeated the same basic errors in the way they trained or tested their tools. “It would have gone a long way to getting the public on our side,” he says. “This pandemic was a big test for AI and medicine,” says Driggs, who is himself working on a machine-learning tool to help doctors during the pandemic.
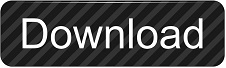